Enrichment: Understanding customers with Speech to Text translation.
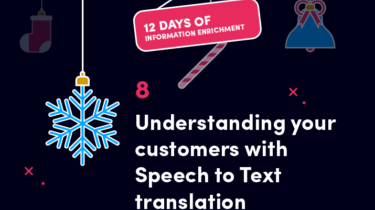
So far in this series, we have talked about enriching text-based content, like Microsoft Word documents and PDF files, and we have spoken about enriching image files with labels that describe what is in the image. But what about enriching voice recordings, such as call centre customer recordings?
Call recording analysis - Why bother?
After all, whenever we ring our insurance company, we are told that ‘this call may be recorded for quality and training purposes'. So, what do these companies do with that information? How do they process it at scale? What insight do they actually derive from it?
The answer is probably not a lot! Not unless they are willing to listen to each call manually, something you are only likely to do in the event of a complaint. Yet, there is tremendous value in these calls. Performing analysis on the calls may help an organisation to understand what makes a successful outcome for their customer. Perhaps it's adding additional cover to an existing policy, or getting a customer to purchase the policy in the first place.
The first step in attempting to do something useful with these calls is being able to transcribe them, to convert them to text so that they are just like any other document. Then, we can process and enrich them.
The challenge of Speech to Text APIs
As part of several customer engagements in this space we have looked at a number of speech to text conversion APIs. The two I am going to briefly talk about here are Google and Microsoft.
The first thing we found is that both APIs are very good at transcribing a single person talking. However, add two people talking on the phone into the mix and things quickly deteriorate. To tackle this problem, we looked at a few options. Many call centre recording systems will record the caller and callee on separate left and right channels. If you have recordings of this nature, this issue with transcribing speech from two different people can be improved quite significantly.
Another challenge is background noise. A notorious problem with call centre recordings, since people will often be in all kinds of situations when making a call to the organisation in question. Again, there are some options in the speech to text APIs that can help smooth this out. However, even when using these advanced options, you will still find quite a lot of words that are transcribed incorrectly.
Getting value from call data
Despite some of these challenges, if we look at the nature of the problems that we are trying to solve, we can still get a lot of value from call transcriptions. This is because we are often only interested in specific indicator words. For example, if we are trying to ascertain if a call to a water provider is about a ‘taste and odour’ complaint, we are going to be interested in quite a specific set of phrases. Of course, these phrases may have been mistranscribed, but Google's API provides a capability that can really help with this. It allows you to configure specific words that Google tries extra hard to get right in the transcription process, by emphasising the detection of these selected words.
So, how does this all fit with InsightMaker? We have enrichment steps that allow us to convert speech to text and store this in the index. We can then leverage all of the downstream enrichment capability we have, such as sentiment analysis or named entity recognition, to enrich the content from the call. We can also utilise our data science steps to perform topic and concept modelling and even classify the calls, for example which ones are complaints and so on. Once the text from the call recording has been enriched, we can start to conduct analysis and begin to answer those crucial business questions.
Overall, speech to text transcription is not without its challenges; you need to be clear on the insight that you are trying to extract from your call data. Having said this, some of the cloud-based APIs for speech to text transcription offer a host of very useful features. Couple this with data science techniques and you can gain valuable insights from your customers' voices.
Cheers and speak soon, Paul
If you missed my blogs in the 12 Days of Information Enrichment series, you can catch up here.
- Day 1 - What is enrichment? Creating wealth from information
- Day 2 - Starting at the beginning with Text Extraction
- Day 3 - Structuring the unstructured with Business Entity Extraction
- Day 4 - Solving the GDPR, PII and PCI problem
- Day 5 - Sustainable Document Classification
- Day 6 - Image Enrichment: Giving your business vision
- Day 7 - Advanced Entity Extraction with Natural Language Processing
- Day 8 - Understanding customers with Speech to Text translation
- Day 9 - Accelerating classification with Document Clustering
- Day 10 - Giving users what they need, when they need it
- Day 11 - Understanding documents with Dynamic Topics
- Day 12 - The power of Enrichment
Stay in the know with updates, articles, and events from Aiimi.
Discover more from Aiimi - we’ll keep you updated with our latest thought leadership, product news, and research reports, direct to your inbox.
You may unsubscribe from these communications at any time. By submitting this form you consent to us processing and storing the information you provide in accordance with our Privacy Policy.
Enjoyed this insight? Share the post with your network.
How to apply AI for Business Intelligence (an ideal first GenAI use case)

Llama 2: Our thoughts on the ground-breaking new large language model
